Algorithms to assess stroke risk are markedly worse for Black Americans
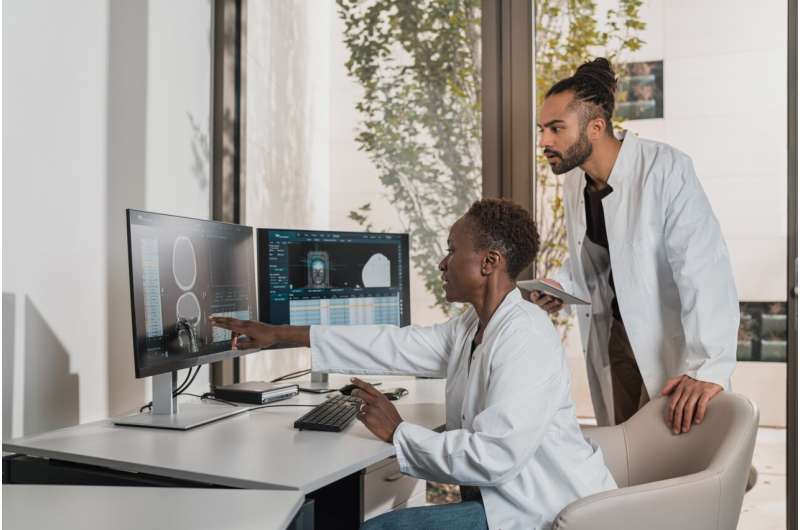
Current medical standards for accessing stroke risk perform worse for Black Americans than they do for white Americans, potentially creating a self-perpetuating driver of health inequities.
一个斯图dy, led by Duke Health researchers and appearing online Jan. 24 in theJournal of the American Medical Association(JAMA), evaluated various existing algorithms and two methods of artificial intelligence assessment that are aimed at predicting a person's risk of stroke within the next 10 years.
The study found that all algorithms were worse at stratifying the risk for people who are Black than people who are white, regardless of the person's gender. The implications are at the individual andpopulation levels: people at high risk of stroke might not receive treatment, and those at low or no risk are unnecessarily treated.
"We need to improvedata collectionprocedures and expand the pool of risk factors for stroke to close the performance gap of algorithms between Black and white adults," said Michael Pencina, Ph.D., corresponding author of the study, professor in the Department of Biostatistics and Bioinformatics and director of AI Health at Duke University School of Medicine.
"For example, the algorithms tested here mostly do not account for social determinants of health and some other factors suggested by the Stroke Prevention Guideline," Pencina said. "Data collection needs to be closer to the patient and the community."
"Disparities can potentially become propagated by these algorithms, and things could get worse for some people, which may lead to inequity in treatment decisions for Black versus white adults," he added.
The study specifically looked at something called risk ordering, which provides perspective on how likely someone is to experience stroke compared to others—an important concept used to allocate limited medical resources.
The study also found that a simple method using answers to patient questions was the most accurate on apopulation leveland that sophisticated machine learning methodologies failed to improve performance.
"While advanced AI techniques have been touted as the most promising path for better algorithms, our results indicate that for simpler types of data like the ones used in our study, complex math does not help," Pencina said.
"The better accuracy of simpler algorithms, based on self-reported risk factors, suggest a promising and potentially cost-effective avenue for preventative efforts," he said.
更多的信息:川港等,预测的准确性中风Risk Prediction Models Across Black and White Race, Sex, and Age Groups,JAMA(2023).DOI: 10.1001/jama.2022.24683