This article has been reviewed according to Science X'seditorial processandpolicies.Editorshave highlighted the following attributes while ensuring the content's credibility:
fact-checked
peer-reviewed publication
trusted source
proofread
New machine learning model improves prediction of prostate cancer recurrence
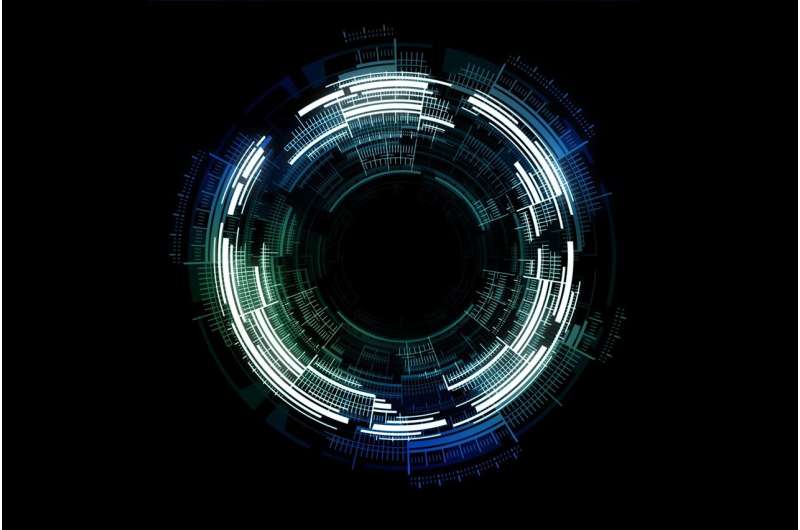
Predicting the course of prostate cancer is challenging because only a fraction of prostate cancer patients experience recurrence after radical prostatectomy or radiation therapy. Yet, prostate cancer remains one of the most fatal malignancies in men in the United States.
Now, investigators have developed amachine learning modelthat combines profiles of fusion genes known to be widespread inprostate cancerwith the commonly used Gleason score andprostate-specific antigen(PSA)水平。食蟹猴的e learning model consistently improved the prediction of prostatecancerrecurrence by theclinical testsalone or in combination. The results are reported inThe American Journal of Pathology.
"Gleason score and PSA level have been used with varying success in predicting clinical outcomes in patients with prostate cancer," explained lead investigator Jian-Hua Luo, MD, Ph.D., Department of Pathology, University of Pittsburgh School of Medicine, Pittsburgh, PA, U.S.. "However, they provide limited insight into the mechanism of the disease. Gene fusion events are known to be widespread in prostate cancer, but their potential in predicting the course of the disease was unknown."
Data from a multi-institutional cohort that included 271 samples ofradical prostatectomyfrom the University of Pennsylvania Medical Center (UPMC), 191 from University of Wisconsin–Madison, and 112 from Stanford Medical Center were analyzed. All 14 of the fusion genes known to be present in prostate cancer were detected in the samples from the combined cohort. Gleason and serum PSA scores were also available.
The investigators first developed a training model using the UPMC data. Several machine learning algorithms were applied to the fusion gene profiling data to determine the best parameters of 14 fusion gene combinations for predicting prostate cancer recurrence. The best algorithms were then applied to the whole training cohort to build a model.
Prediction of cancer recurrence based on Gleason score alone had 77.9% accuracy, and PSA alone correctly predicted 73.5% of prostate cancer recurrence. When the Gleason score data were incorporated in the machine learning analysis with the fusion data, a total of 442 models of different combinations showed an accuracy above 80% for the combined models.
When PSA alone was combined with fusion data, 265 models of different combinations showed prediction rates above 75%. The combination of fusion data, Gleason score, and PSA improved the prediction of prostate cancer; 317 models yielded prediction rates of 80% or better.
Next, 764 machine learning models trained using data from the UPMC cohort were applied to the Stanford/Wisconsin cohort, and then to the UPMC/Stanford/Wisconsin cohort. Again, the combination of fusion data, Gleason score, and PSA outperformed the prediction of cancer recurrence by PSA or Gleason score alone or combined.
Cancer did not recur for five years after surgery in 81.9% of patients if the cancer was predicted as nonrecurrent, while only 17.2% of patients were recurrence free if their cancer was predicted as recurrent by the same model. With the Gleason plus PSA model, 78.3% of patients had no cancer recurrence if the cancer was predicted as nonrecurrent by the model, and 26.2% of patients had no cancer recurrence for five years if the cancer was predicted as recurrent.
Dr. Luo notes that profiles of fusion gene have added value for clinical patient management because some gene fusions are important molecular processes in generating prostate cancer, whereas others are known to make cancer susceptible to certain drugs. "One of the big surprises in the analysis is that the fusion genes called CCNH-C5orf30 turned out to be an indicator of favorable clinical outcomes. It is unusual for a genomic abnormality created bycancer cellsto restrain cancer's aggressiveness," he said.
"The detection offusion genesprovides new mechanistic insight into prostate cancer progression enabling proactive measures to be taken," Dr. Luo observed. "The incorporation of fusion gene detection into theprostatecancer diagnostic scheme benefits patients with regard to diagnosis, prognosis, cancer progression surveillance, and treatment. Further, if these machine learning models are applied toclinical practicein the future, more lives may be saved."
更多的信息:Yan-Ping Yu et al, Fusion Gene Detection in Prostate Cancer Samples Enhances the Prediction of Prostate Cancer Clinical Outcomes from Radical Prostatectomy through Machine Learning in a Multi-Institutional Analysis,The American Journal of Pathology(2023).DOI: 10.1016/j.ajpath.2022.12.013